Enhanced Decision Making With Alternative Data- Decisimo - Decision Intelligence Services
Published on: 2024-08-10 18:48:28
“Garbage in, garbage out. Or rather more felicitously: the tree of nonsense is watered with error, and from its branches swing the pumpkins of disaster.”
This quote from the novel “The Gone-Away World” is spot-on for the world of decision making too...
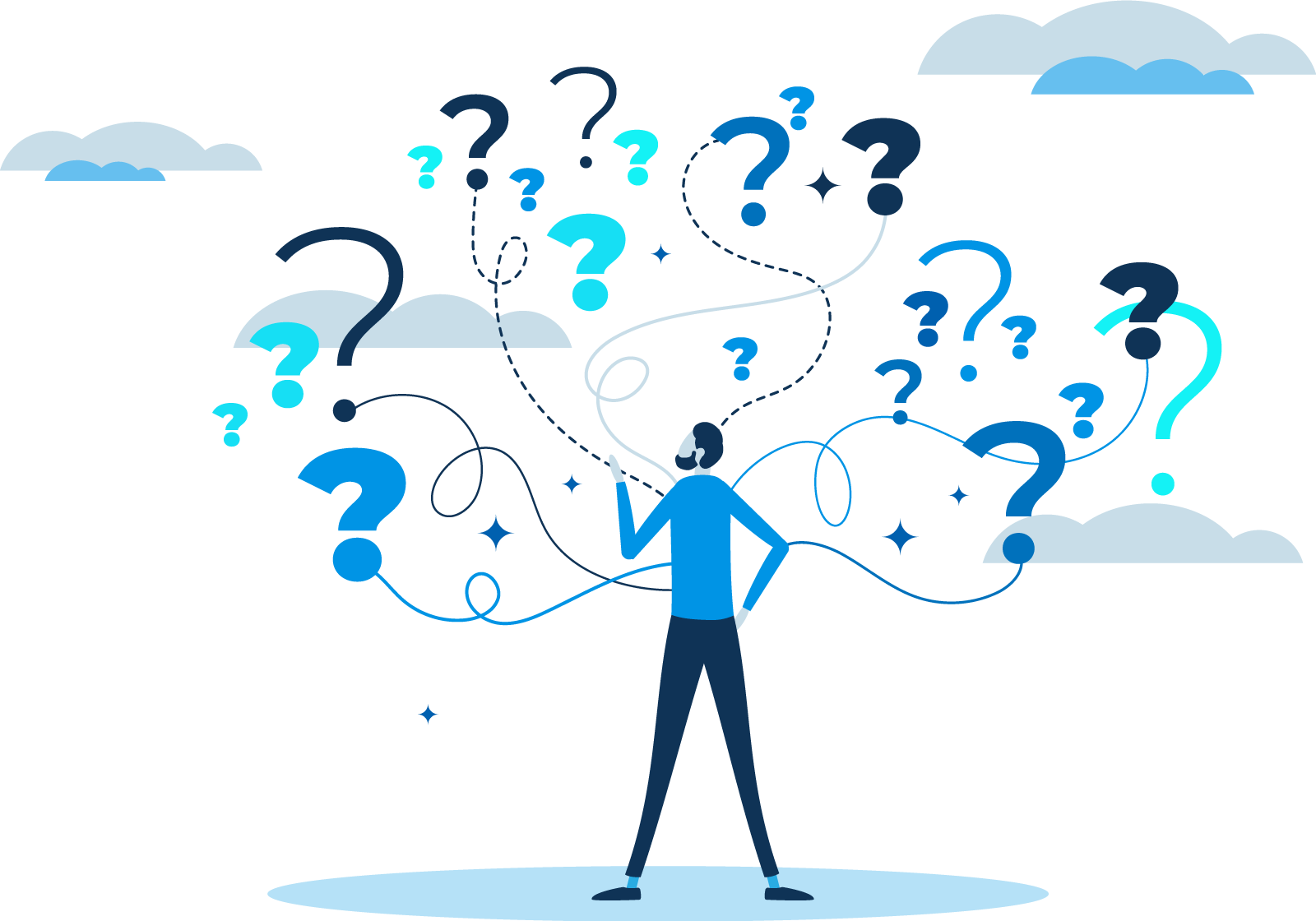
The authenticity and integrity of the data used in the decision making process, will determine the quality of the decisions made. It also influences the desired outcomes. Data sources include 2 categories: primary and secondary.
The primary data source is from the customer/user himself inputting data and answering questions in the dataflow. Secondary sources include alternative data providers/data brokers. If less data is collected from the customer/primary source, more data has to be collected or augmented from secondary sources/brokers.
The type of data for enrichment can be traditional personal identifiers, like phone numbers, email addresses, and physical addresses. More technical data, like device information, browser data, IP addresses, etc. can also be enriched.
Assisted login data collection
Data brokers’ data sources include API requests and “assisted login data collection”. API requests are very straightforward: send requests and receive data through the responses. “Assisted login data collection” on the other hand, poses some challenges to data quality and integrity.
Assisted login data collection can vary from Open Banking API standards to account scraping. Account scraping often occurs when users provide login credentials on a site. In the background, data providers log into the data and “scrapes” it. They clean it, and send it to the “data consumer” (the business where the user is providing the credentials). Some companies/websites try to prevent scraping and no data will be collected in such cases.
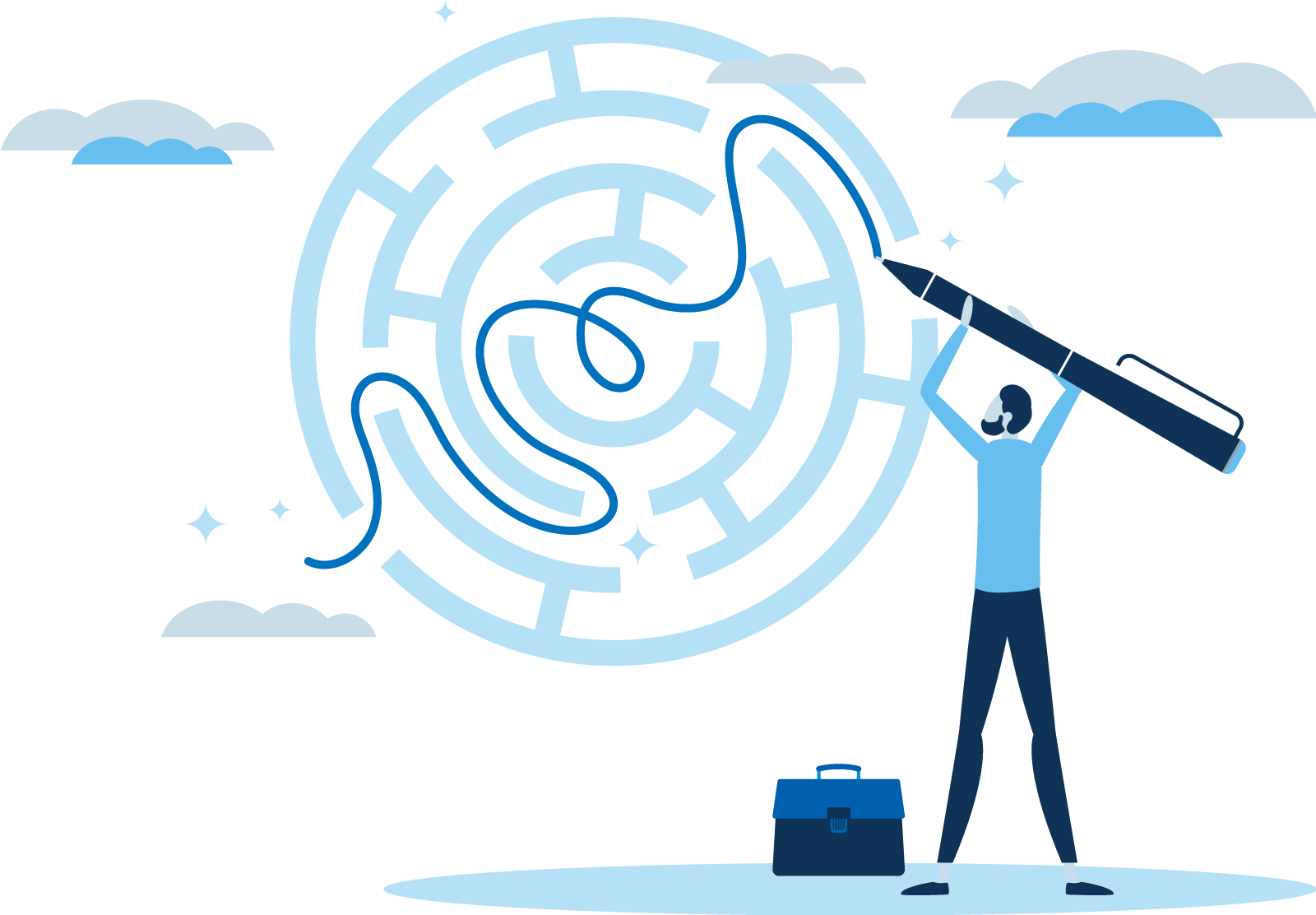
Challenges to alternative data sourcing
Due to the nature of the collection of the alternative data and how it is processed, the data can be incomplete. The data quality can be dubious and lack the integrity required to be useful. Sometimes the whole service can be unavailable – that happens when the “scraped” site is preventing data collection.
Traditionally, alternative data is used for the purpose of better segmentation and customer profiling for marketing. It is used to train predictive credit scoring models, towards enriching user profiles and to verify user identity. Fraud and data misuse can be minimized too.
Alternative data sourcing faces two key challenges: legality and outages. Although the customer is the owner of the information, platforms that are being scraped are opposed to it. Furthermore, they are actively preventing it and even calling it data “theft”.
Another challenge is service outages that result in technical errors of “service unavailable”. Data responses may be missing. Though they appear to be successful: some values are missing, and data is incomplete. Response times are occasionally longer than business processes allow. This makes it impossible to gather the desired data successfully.
With this background in mind, there are some good practices and controls that can be put into place to help when it comes to alternative data:
- Expect that there will be data problems.
- Build robust decision flows that execute even with missing data.
- When using multiple data sources, call them in parallel to reduce execution time.
- Cache for the period that you are sure the data could not have changed from the business point of view. Sometimes just asking the source – how often do you refresh? can be helpful.
- When building predictive models and categorizing variables, separate and differentiate between “no response”, “no data” and “null value” – each has a different business meaning and can have different predictive power. By combining it into a one single “null value”, you can lose a lot of valuable “predictiveness”.
Making use of alternative data sources does not have to influence the quality of the decision making process and its outcome adversely. Certain safeguards can be implemented to prevent the “garbage in; garbage out” scenario.